The problem with serverless scaling
In the first article, I wrote about our live statistics system and why we implemented it on our website. In later articles, I want to dive deep into problems we have had with scaling the system to a growing number of users and what we did to tackle chosen problems.
Releasing the feature to the public
After putting the product/feature on our website, our first broadcast/event occurred. It was the Fall Final 2022 event in November, and it was the first time we would show our users/fans the new real-time stats feature we have built over the last five weeks. We were stoked to see what the fans were thinking about it and how they would react.

At the start, we saw around 3-400 people watching the live stream on our website, and everything was running smoothly, with no errors occurring yet. The viewership grew with around a thousand people, and we started to see errors, and users reporting that the stats weren’t working for them.
We tried to investigate if either the API or database was failing. It wasn’t the API that was struggling, because we chose to build the API upon the Lambda serverless function infrastructure on AWS, which could scale to 1500 running instances at once. Then we looked at how the database was handling the traffic, and it was struggling, with 1000 connections to the database.
Pain with serverless computing scaling
After the event, we sat down to reflect on what caused the outage of real-time stats. We wrote a postmortem about what happened and investigated how we can resolve this issue so that in the future, we can scale this feature to more people than we had in this event (around 1200 people).
The first place we started looking for issues was CloudWatch. There was a similarity in the metrics tabs of both the database and the API between Lambda concurrent executions and database connections.
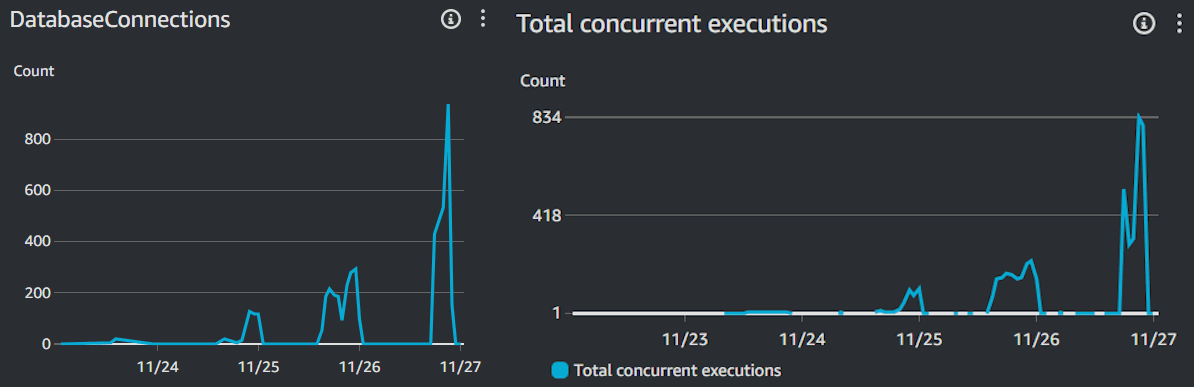
We could see that we had a lot of concurrent executions on the last day of the event, which made sense because a lot of people tuned in to watch the grand final and the showmatch on BLAST.tv
By looking at the metrics we could conclude that for every container that lambda spun up, it would generate a connection to the database, which meant that we needed to implement some API caching or reduce the amount of API calls that were made by every user each x second to the API which returns the live stats.
Database Proxy implementation
The current API implementation meant that each single one of the viewers would send a GET request to the statistics API, which would query the data and return it to the user. For every x amount of seconds we specified, we would make each one of the users request the stats. So this meant if we had 1500 concurrent viewers on our live stream, we would have 1500 requests every 15 seconds.
We would see the requests coming in waves because the interval/timer starts when the user loads the live page. Our database wasn’t happy with 1500 heavy queries every 15-20 seconds, even though the database can scale to 128 ACUs.
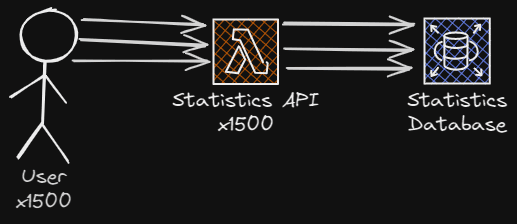
The database was struggling because of the amount of connections to the database, so we tried setting up a database proxy in front of it, in the hope that it could reduce the load on the database.
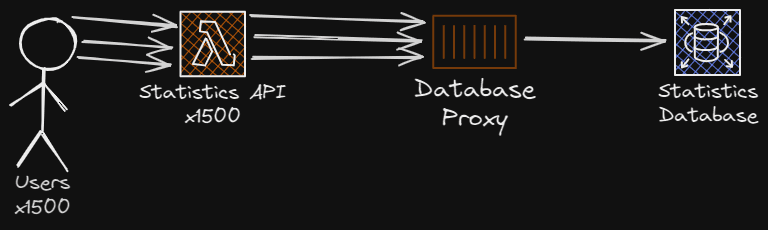
The proxy would be in front of the database to handle database pooling for us, so when the lambdas scale to hundreds of instances, they would connect to the database proxy, which would handle the database connections and the transactions to the actual database.
The database proxy would allow us to scale the lambdas and keep the number of connections to the database below a fixed threshold so the database wouldn’t crash. We can do this, because the database proxy helps us pooling the connections to the database, and allows the connections to be re-used for queries.
Bug fixing under stressful situations
We tried implementing the database proxy during the actual event when the problem occurred after deploying what we thought was the fix with the database proxy, but it didn’t seem to have changed regarding the database connections. The connections were still around the ~800 mark.
After the event was over, and we tried our best to survive the rest of the days during the event, we sat down to try to figure out why our changes with the database proxy we deployed didn’t work. After some research, we found that we missed linking the lambda function with the database proxy.
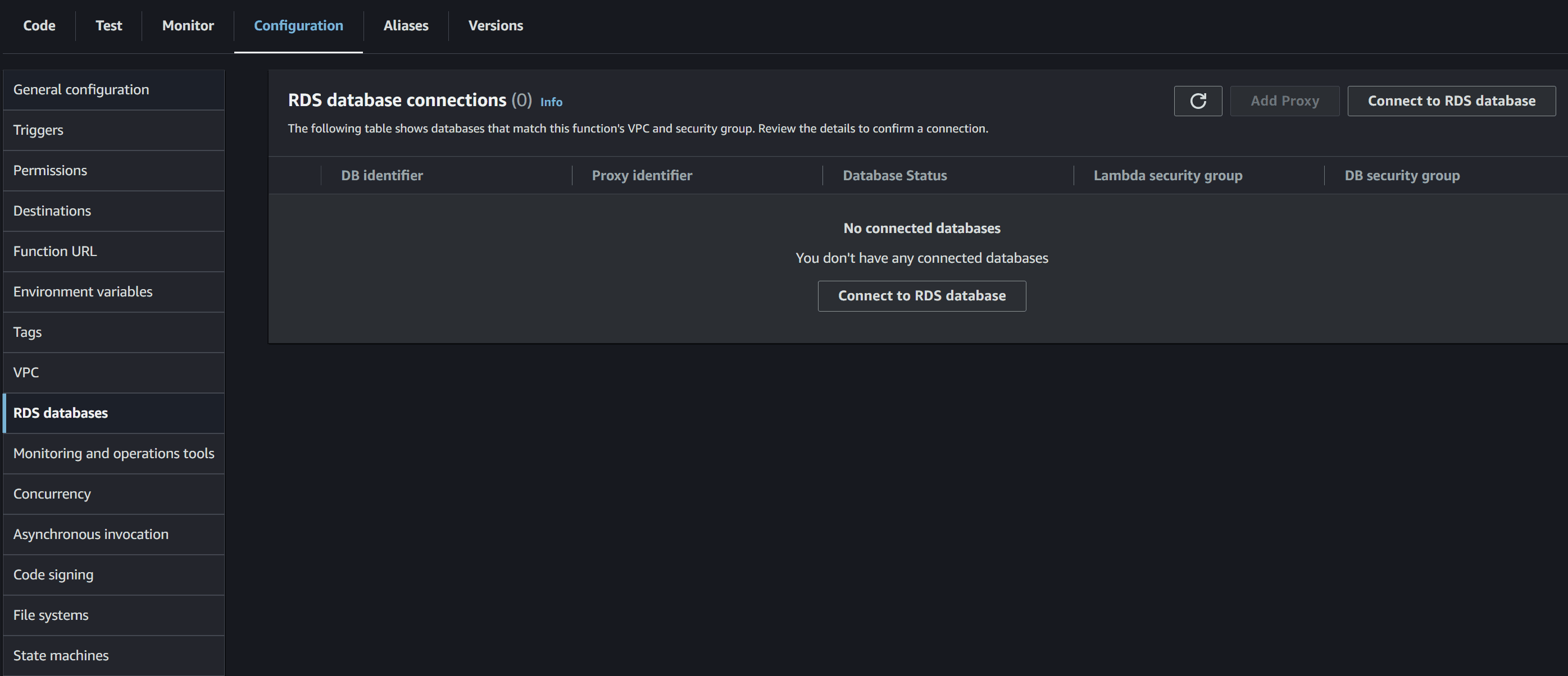
There is a setting under the lambda function, which you can select the database or database proxy it would connect to, which would reduce the CPU and memory usage of our database and handle the database connection automatically from the lambda function to the database proxy.
Conclusion
There are a few points the BLAST.tv team learned during this outage of Live Statistics during Fall Final 2022. First and foremost, we needed to have load-tested the system before launching it. We had only tested it on our development environment (with a maximum of 5 users), which resulted in us not knowing how the system would scale to hundreds of users during a live event.
The second learning we can take away from this would be even though we are in a stressful situation we shouldn’t panic and start deploying all sorts of things. It’s better to sit down, breathe a little, and find which solutions are available and how to implement them. It’s also better to deploy a solution to a development environment so you can test the solution before promoting it to production. Doing this would minimize the amount of downtime of a given service/system. It’s also a good idea to deploy a single solution one at a time. That way, can you ensure that you can test if the solution fixes the problem and tell what worked and what didn’t work.
Thank you for reading through this article. In the later articles, I want to explain why we didn’t go with API caching and the different types of caching methods there are.